The use of AI in financial markets for algorithmic trading and fraud detection
- By Tunzeel Ahmed
- 21-02-2023
- Artificial Intelligence
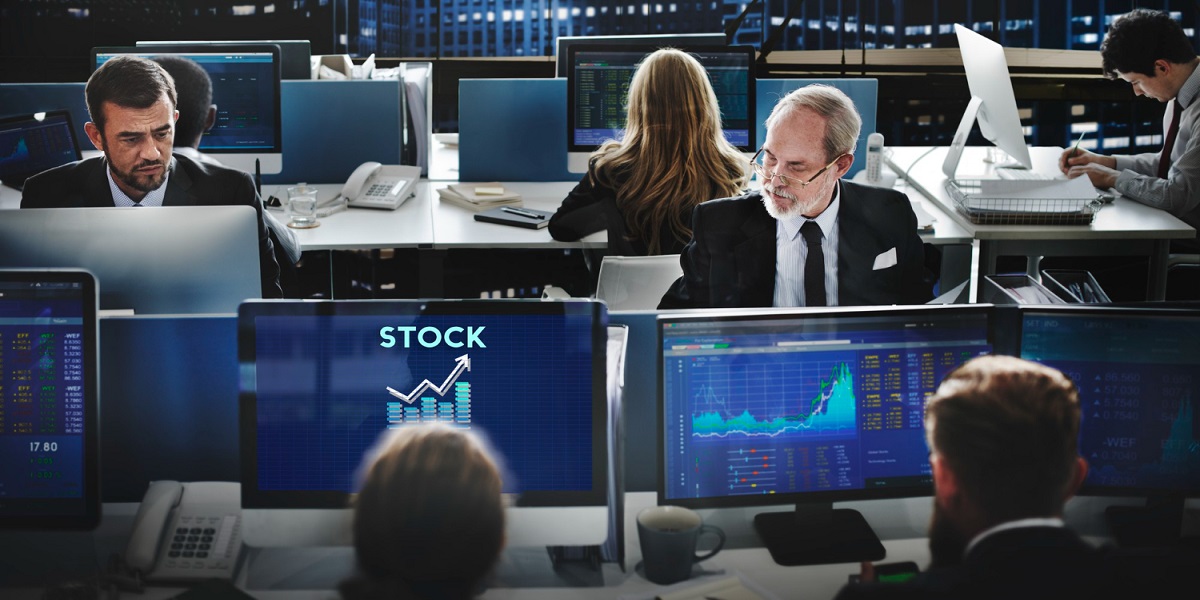
AI is increasingly being used in financial markets for a variety of tasks, including algorithmic trading and fraud detection. Algorithmic trading, also known as "algo-trading" or simply "trading," involves the use of computer programs and algorithms to automatically execute trades on financial markets. These algorithms can analyze large amounts of data and make trades based on predefined rules and patterns, allowing for more efficient and effective trading.
Fraud detection is another area where AI is being used in the financial sector. Machine learning algorithms can be trained to identify unusual patterns or anomalies in financial transactions that may indicate fraudulent activity. By analyzing large amounts of data in real-time, these systems can detect potential fraud more quickly and accurately than traditional methods.
However, there is also concerns about the use of AI in financial markets, such as the potential for errors or biases in the algorithms, the possibility of unintended consequences and the need for proper regulation.
Use of AI ghostwriting services
AI ghostwriting services are companies or platforms that offer to generate written content using AI-powered tools and software. These services typically use natural language processing (NLP) and machine learning algorithms to analyze existing text and generate new text that is similar in style and content. AI ghostwriting services can be used to create written content in a variety of fields, such as journalism, marketing, and even literature.
Some examples of AI ghostwriting services include:
- Article writing services: These services use AI algorithms to generate news articles, blog posts, and other written content on a variety of topics.
- Social media post writing services: These services use AI algorithms to generate social media posts for businesses and individuals.
- Email writing services: These services use AI algorithms to generate professional emails for businesses and organizations.
- Content creation for e-commerce: These services use AI algorithms to generate product descriptions, product reviews, and other written content for e-commerce websites.
- Copywriting services: These services use AI algorithms to generate persuasive and compelling copy for advertising, marketing and branding purposes.
AI ghostwriting services can be a useful tool for businesses, organizations, and individuals who need to generate written content quickly and cost-effectively. However, it's important to note that the quality of the content generated by these services may not be as high as that created by human writers. And there are also ethical concerns such as potential for AI-generated content to deceive readers or misrepresent the views of an organization or individual. It's important to be aware of these limitations and make sure to use these services appropriately.
Explanation of algorithmic trading and how AI is used
Algorithmic trading, also known as "algo-trading" or simply "trading," is the use of computer programs and algorithms to automatically execute trades on financial markets. The algorithms used in algorithmic trading are designed to analyze large amounts of data and make trades based on predefined rules and patterns.
AI techniques such as machine learning and natural language processing are commonly used in algorithmic trading to analyze market data, identify patterns and make predictions about future market movements. These algorithms can be trained on historical data to identify patterns and predict future trends, and then use this information to make trades. Some of the AI techniques used in algorithmic trading include:
Machine learning algorithms, which can be trained on historical market data to identify patterns and predict future market movements.
Natural language processing (NLP) algorithms, which can be used to analyze news articles and social media posts to gain insight into market sentiment.
Predictive modeling, which uses historical data to predict future market movements.
Algorithmic trading can also be used to execute trades based on these predictions, which enables traders to make trades faster and with more precision than they would be able to do manually. However, as with any technology, it also comes with certain risks such as errors in the algorithms, lack of transparency, and the need for proper regulation.
Advantages of using AI in algorithmic trading
There are several advantages to using AI in algorithmic trading, including:
- Speed and Efficiency: AI-powered algorithms can analyze large amounts of data quickly and make trades based on that analysis in real-time, which allows for faster and more efficient trading.
- Improved Accuracy: By analyzing historical data, AI algorithms can identify patterns and predict future market movements with a high degree of accuracy. This can help traders make more informed decisions and potentially increase their returns.
- 24/7 Trading: AI algorithms can execute trades automatically, which means they can continue trading even when human traders are not available.
- Cost-effectiveness: AI-driven algorithms can help traders to reduce costs associated with human traders, such as wages and benefits.
- Automation: AI algorithms can automate repetitive tasks such as data collection and analysis, which frees up traders to focus on more complex and higher-level tasks.
- Risk Management: AI algorithms can help traders to identify and manage risks by analyzing large amounts of data and identifying patterns that may indicate potential risks.
- Scalability: AI algorithms can handle large amounts of data, which means they can be used to trade in multiple markets and with different assets.
Examples of AI-powered trading systems
There are several examples of AI-powered trading systems that are currently being used in the financial markets, including:
- High-Frequency Trading (HFT) systems: These systems use complex algorithms and high-speed computer networks to execute trades at lightning-fast speeds. Some HFT systems use AI techniques such as machine learning to analyze market data and make trades based on that analysis.
- Robo-Advisors: Robo-advisors are AI-powered investment management systems that use algorithms to create and manage investment portfolios for individual investors. These systems use data on an individual's investment goals, risk tolerance, and other factors to create a customized investment strategy.
- Sentiment Analysis: This AI-based system analyzes news articles and social media posts to gain insight into market sentiment, which can be used to predict future market movements.
- Predictive modeling: This system uses historical data to predict future market movements and make trades accordingly. Predictive models can be trained to recognize patterns and trends in the data, and then use this information to make predictions about future market movements.
- Portfolio Optimization: This system uses AI algorithms to optimize a portfolio by selecting the best performing assets and reallocating them to achieve a desired level of risk and return.
- Risk Management: AI algorithms can help to identify and manage risks by analyzing large amounts of data and identifying patterns that may indicate potential risks, such as an unusually high volume of trades in a particular security.
Explanation of how AI is used in fraud detection in financial markets
In the financial markets, AI-based fraud detection systems use machine learning algorithms to identify unusual patterns or anomalies in financial transactions that may indicate fraudulent activity. These systems can be trained on historical data to learn the patterns of fraudulent activity and can then use this information to detect similar patterns in real-time transactions.
There are several steps in the process of using AI for fraud detection in financial markets:
Data collection: The first step is to collect historical data on financial transactions, including information on both fraudulent and non-fraudulent transactions. This data is used to train the machine learning algorithms.
- Data preprocessing: The collected data is then cleaned and preprocessed to prepare it for analysis. This step involves removing any irrelevant data, filling in missing values and transforming the data into a format that can be easily analyzed by the machine learning algorithms.
- Model training: The machine learning algorithms are then trained on the preprocessed data. The algorithms learn to identify patterns and correlations in the data that are indicative of fraudulent activity.
- Model deployment: Once the model has been trained, it can be deployed in a production environment to detect fraudulent activity in real-time transactions.
- Real-time monitoring: The system continuously monitors financial transactions and flags any transactions that match the patterns of fraudulent activity that the system has been trained to recognize.
- Evaluation and fine-tuning: The system's performance is continuously evaluated and fine-tuned to improve its accuracy and reduce the number of false positives.
AI-based fraud detection systems can help financial institutions to detect fraud more quickly and accurately than traditional methods. However, as with any technology, it also comes with certain risks such as errors and biases in the algorithms, lack of transparency, and the need for proper regulation.
Advantages of using AI in fraud detection
There are several advantages to using AI in fraud detection, including:
- Speed and Efficiency: AI-powered algorithms can analyze large amounts of data quickly and identify fraudulent activity in real-time, which allows for faster detection of fraudulent activity.
- Improved Accuracy: By analyzing historical data, AI algorithms can identify patterns and correlations in the data that are indicative of fraudulent activity, which can lead to a higher rate of detection of fraudulent activity.
- Scalability: AI algorithms can handle large amounts of data, which means they can be used to detect fraudulent activity in multiple markets and with different types of transactions.
- Automation: AI algorithms can automate repetitive tasks such as data collection and analysis, which frees up human analysts to focus on more complex and higher-level tasks.
- Adaptability: AI algorithms can be updated and fine-tuned to adapt to new types of fraudulent activity, which helps to keep fraud detection systems up-to-date.
- Risk Management: AI algorithms can be used to identify and manage risks by analyzing large amounts of data and identifying patterns that may indicate potential risks.
- Reducing human error: AI-based systems can reduce human error and the potential for human bias in identifying fraudulent activity.
Examples of AI-powered fraud detection systems
There are several examples of AI-powered fraud detection systems currently being used in the financial markets, including:
- Transaction monitoring systems: These systems analyze financial transactions in real-time and flag any transactions that match the patterns of fraudulent activity that the system has been trained to recognize.
- Anomaly detection systems: These systems use machine learning algorithms to identify unusual patterns or anomalies in financial transactions that may indicate fraudulent activity.
- Behavioral analysis systems: These systems analyze the behavior of customers and flag any unusual or suspicious behavior that may indicate fraudulent activity.
- Natural Language Processing (NLP) based systems: NLP algorithms can be used to analyze text data such as emails, chats, and social media posts to detect fraud by identifying patterns of suspicious language or behavior.
- Hybrid systems: These systems use a combination of different AI-based techniques such as rule-based systems, statistical models, and machine learning to detect fraud.
- Deep learning-based systems: These systems use neural networks to analyze large amounts of data and detect patterns that indicate fraudulent activity.
- Graph-based systems: These systems use graph theory and network analysis to detect fraud by identifying patterns of fraudulent activity in a network of financial transactions.
Discussion of challenges and limitations of using AI in financial markets
There are several challenges and limitations when using AI in financial markets, including:
- Data quality and availability: To train and deploy AI-based systems, large amounts of high-quality data are required. However, in some cases, the data may be incomplete, inaccurate, or biased, which can affect the performance of the AI-based systems.
- Lack of transparency: Some AI-based systems, particularly those using deep learning techniques, can be difficult to interpret and understand, which can make it challenging to explain the system's decisions and identify errors or biases.
- Model overfitting: AI-based systems can be prone to overfitting, which occurs when the model is too closely fit to the training data and performs poorly on new data.
- Lack of interpretability: Some AI-based systems can be difficult to interpret and understand, which makes it challenging to explain the system's decisions and identify errors or biases.
- Ethical concerns: AI-based systems can perpetuate biases present in the data, and may also be used in ways that are not in the best interest of society, such as manipulation of financial markets.
- Lack of regulation: The use of AI in financial markets is a relatively new field and there is a lack of regulation in place to govern the use of AI-based systems in this sector, which can raise concerns about transparency, accountability, and security.
- Cybersecurity: AI-based systems can be vulnerable to cyber attacks, which can compromise the security and integrity of the financial markets.
- Dependence on technology: AI-based systems can be complex and depend on technology, which can create a risk of system failure or malfunction.
Ethical and regulatory considerations
The use of AI in financial markets raises several ethical and regulatory considerations, including:
- Bias: AI-based systems can perpetuate biases present in the data, which can lead to unfair or discriminatory outcomes. For example, a machine learning algorithm that is trained on historical data that is biased against certain groups of people may make biased predictions or decisions.
- Transparency: Some AI-based systems, particularly those using deep learning techniques, can be difficult to interpret and understand, which can make it challenging to explain the system's decisions and identify errors or biases.
- Accountability: AI-based systems can make decisions that have significant financial and legal consequences, but it can be difficult to hold the individuals or organizations responsible for those decisions accountable.
- Privacy: AI-based systems can collect and analyze large amounts of personal data, which raises concerns about data privacy and security.
- Fairness: AI-based systems can be used to make decisions that have significant consequences for individuals and groups, such as loan applications or insurance claims. Ensuring that these decisions are fair and unbiased is crucial.
- Governance: The use of AI in financial markets is a relatively new field and there is a lack of regulation in place to govern the use of AI-based systems in this sector, which can raise concerns about transparency, accountability, and security.
- Explainability: AI-based systems can make decisions that are difficult to explain, which can make it difficult to understand the system's reasoning and identify errors or biases.
- Ethical use: AI-based systems can be used in ways that are not in the best interest of society, such as manipulation of financial markets.
To address these ethical and regulatory considerations, financial institutions and regulators may need to develop new policies and regulations to govern the use of AI in financial markets, as well as implement measures to ensure transparency, accountability, and fairness. Additionally, financial institutions may need to invest in developing explainable AI systems and ensure that the systems are subject to regular audits and evaluations.